Introduction
The rise of artificial intelligence (AI) has brought the term “prompting” into mainstream conversations. Prompting, the act of giving instructions to AI, is often perceived as a modern skill. However, the practice of human-computer interfacing is deeply rooted in history, dating back to the earliest programmable machines. Charles Babbage’s Analytical Engine (1837) stands as one of the earliest examples of mechanical computation, where structured inputs were necessary to produce logical outputs. Babbage’s collaborator, Ada Lovelace, conceptualised the first algorithm for this machine, laying the groundwork for programming as a discipline. Later, Alan Turing’s theoretical Turing Machine formalised the concept of computation, showcasing the power of algorithms to solve abstract problems (Turing, 1936).
These milestones emphasise one fundamental truth: clear, structured communication is the cornerstone of effective machine interaction. Today, generative AI tools such as ChatGPT provide a façade of simplicity, suggesting that anyone can use AI to solve problems. Yet, without understanding how these tools process inputs, outputs often fail to meet expectations. Engineers who understand pseudo code—an intermediate step between natural language and formal programming—are uniquely positioned to bridge this gap. By leveraging pseudo code, they can translate their ideas into machine-readable logic, ensuring their prompts produce precise and meaningful results.
Historical Foundations of Human-Computer Interaction
The foundation of human-computer interaction lies in cybernetics, a field pioneered by Norbert Wiener (1948), which explored feedback loops in communication systems. Wiener’s work highlighted the importance of structured communication, laying the foundation for modern algorithms. Asimov’s “Three Laws of Robotics” (1942) underscored the need for precise instructions, ensuring machines operated safely and predictably. However, these principles have limitations. Der Derian (2010) criticises their application in autonomous warfare, where vague instructions can result in unintended consequences.
Structured programming, introduced by Edsger Dijkstra, further solidified the importance of logical design in machine communication (Dijkstra, 1968). These historical developments highlight the critical role of precision in reducing errors and improving outcomes in human-machine interactions.
The Convergence of Disciplines
Engineering has undergone significant transformations, with the boundaries between mechanical and computer engineering increasingly blurred. CAD systems, 3D printing, and digital twins have revolutionised prototyping, enabling faster and more efficient designs (Tao et al., 2018). AI now plays a pivotal role in optimising these systems, generating simulations, and predicting performance.
For example, creating a digital twin requires defining variables such as dimensions, material properties, and constraints. Without pseudo code, engineers risk generating incomplete or incorrect models, leading to inefficiencies and delays. Structured prompts in pseudo code ensure that AI interprets these inputs correctly, bridging the gap between human intent and machine execution.
Teaching Pseudo Code: The Theory Behind Structured Programming
Foundations of Structured Programming
Pseudo code simplifies complex logic into step-by-step instructions using control structures that underpin all programming languages:
- Sequential Execution: Instructions are executed in the order they appear.
- Conditional Statements: Decision-making processes using
if-then
orif-then-else
. - Loops (Iteration): Repeated execution of code blocks, such as
while
,do-while
, orfor
.
These principles enable users to articulate problems clearly, making them accessible to both humans and machines.
Pseudo Code in Action: Programming a Robot for Combat
Consider a scenario where two wheeled robots compete in a “Robot Wars”-style arena. The goal is to program a robot to defeat its opponent using strategy and precision.
Example of a Poorly Structured Prompt:
“Make a robot that fights effectively and wins the battle.”
- AI Output: A generalised script, e.g., “The robot moves randomly and attacks when in range.” This lacks strategy, leading to inefficiencies and poor performance.
Example Using Pseudo Code:
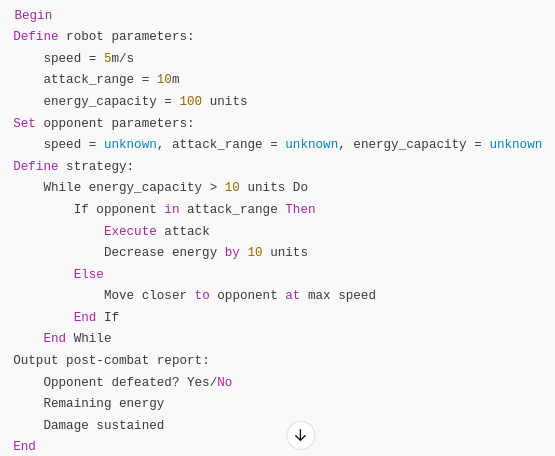
- AI Output: A refined strategy where the robot optimises its movements, manages energy efficiently, and attacks only when necessary.
Applications in Manufacturing and Industry 4.0/5.0
The emergence of Industry 4.0, characterised by the integration of IoT, AI, and automation, has transformed manufacturing processes by enabling smarter, interconnected systems. Building on this foundation, Industry 5.0 emphasises human-AI collaboration, aiming to create more sustainable and human-centric approaches to production. Within this landscape, pseudo code serves as a critical tool, allowing engineers to communicate their design requirements clearly and precisely, thereby enhancing productivity and reducing errors.
Prototyping and Optimisation
Prototyping is a cornerstone of manufacturing innovation, and the advent of digital twins has further revolutionised this process. A digital twin—a virtual replica of a physical system—allows engineers to test designs in a simulated environment before committing to physical prototypes. Using pseudo code, engineers can define key parameters such as torque, speed, payload capacity, and environmental conditions, ensuring that the digital twin reflects real-world constraints accurately.
For example, designing a robotic arm for assembly requires engineers to balance factors like joint flexibility, operational speed, and load distribution. Without pseudo code, engineers might rely on iterative trial-and-error methods, leading to increased costs and extended development times. By specifying these variables in pseudo code, engineers provide AI with the structured inputs needed to generate optimised digital twin models, significantly reducing physical testing requirements and accelerating production timelines (Tao et al., 2018).
Moreover, pseudo code facilitates automated optimisation processes. By incorporating conditional logic and iterative loops, engineers can instruct AI systems to test multiple configurations, identify inefficiencies, and suggest improvements. This iterative refinement process, powered by pseudo code, enables manufacturers to achieve better performance metrics while minimising waste and resource consumption.
Robotics Programming
Industrial robots play an essential role in modern manufacturing, performing tasks such as welding, assembly, material handling, and quality inspection. However, programming these robots to execute tasks safely and efficiently requires meticulous planning. Pseudo code provides engineers with a means to articulate complex task sequences, safety protocols, and contingency plans in a clear and logical format.
Consider a welding robot tasked with joining components along a production line. Using pseudo code, an engineer can define the robot’s movement paths, welding parameters (e.g., temperature and speed), and error-detection mechanisms.
Reducing Interface Errors
AI’s ability to interpret human input is inherently constrained by its training data, which cannot encompass every possible scenario (Floridi et al., 2018). Ambiguity in communication often leads to outputs that fail to meet user expectations, resulting in wasted time and resources. Pseudo code addresses this challenge by providing a structured framework for interaction, reducing the likelihood of misinterpretation.
Conclusion
Pseudo code bridges the gap between human logic and machine execution, enabling engineers to leverage AI effectively. By articulating precise instructions, engineers can optimise workflows, reduce errors, and achieve better outcomes in applications ranging from robotics to manufacturing. The future of engineering lies at the intersection of human expertise and AI capabilities, and pseudo code is the tool that will guide this collaboration into the next industrial era.
References
- Asimov, I. (1942). Runaround. In I, Robot. Gnome Press.
- Babbage, C. (1837). On the Analytical Engine. British Museum Archive.
- Dijkstra, E. (1968). “Go To Statement Considered Harmful.” Communications of the ACM, 11(3), 147–148.
- Der Derian, J. (2010). Virtuous War: Mapping the Military-Industrial-Media-Entertainment Network. Routledge.
- Floridi, L., et al. (2018). “AI as Augmented Intelligence: Beyond Machine Learning.” Philosophy & Technology, 31(4), 317–328.
- Goldberg, D. (1989). Genetic Algorithms in Search, Optimization, and Machine Learning. Addison-Wesley.
- Knuth, D. (1974). The Art of Computer Programming: Fundamental Algorithms. Addison-Wesley.
- Tao, F., et al. (2018). “Digital Twin and Smart Manufacturing.” Advanced Engineering Informatics, 39, 845–856.
- Turing, A. (1936). “On Computable Numbers, with an Application to the Entscheidungsproblem.” Proceedings of the London Mathematical Society, 42(1), 230–265.
- Wiener, N. (1948). Cybernetics: Or Control and Communication in the Animal and the Machine. MIT Press.